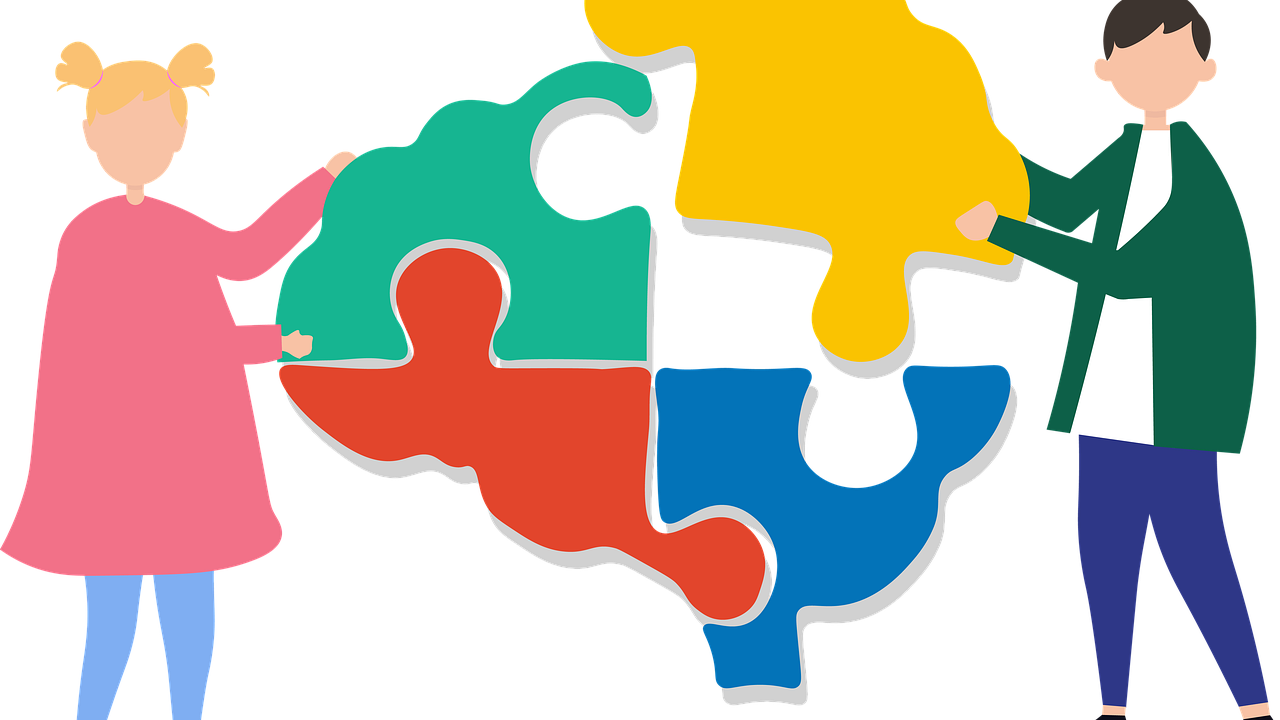
New Computation Model to Help Diagnose Autism
- News
- 1.4K
Improved diagnosis of neurodevelopmental disorders, better road safety while driving, and enhanced reliability of eyewitness testimonies could be in the offing with the development of a new computational model that can predict a person’s ability to detect changes in the visual environment.
Human brains have a remarkable ability to pay attention to details but may sometimes fail to notice even marked differences. This phenomenon of overlooking a visual change is called change blindness. It is of particular importance for improving the reliability of the testimonies of eyewitnesses, among other things.
A research group at the Centre for Neuroscience and the Department of Computer Science and Automation at the Bengaluru-based Indian Institute of Science (IISc) has been studying the issue and has come up with a computational model of eye movement that can predict a person’s ability to detect changes in the visual environment.
In the study, the team first checked for change blindness among 39 people by showing them an alternately flashing pair of images that have a minor difference between them. “We expected some complex differences in eye movement patterns between subjects who could do the task well and those who could not. Instead, we found some very simple gaze-metrics that could predict the success of change detection,” recounts Sridharan Devarajan, Associate Professor at the Centre for Neuroscience and a member of the research team.
Successful change detection was found to be linked to two metrics: how long the subjects’ gaze was fixated at a point, and the variability in the path taken by their gaze between two specific points. Subjects who fixated for longer at a particular spot, and whose eye movements were less variable were found to detect changes more effectively.
Other researchers have previously developed models that focus either only on eye movement or on change detection. The new model goes one step further. It combines both aspects.
The researchers tested their model against a state-of-the-art deep neural network called DeepGaze II and found that their model performed better than it at predicting human gaze patterns in free-viewing conditions – when the subjects were casually viewing the images. While DeepGaze II could predict where a person will look if presented with an image, it did not work as well as the IISc-developed model at predicting the eye movement pattern of a person searching for a difference in the images. “It’s not enough to just predict where a subject will look, the model also has to take into consideration the goals of the subject when they view images,” explains Sridharan.
In the future, the researchers plan to incorporate artificial neural networks with “memory” into the model – to more realistically mimic the way our brains retain recollections of past events to detect changes.
The authors noted that the insights into understanding change blindness provided by their model could help scientists better understand visual attention and its limitations and said that some examples of areas where such insights can be applied include diagnosing neurodevelopmental disorders like autism, improving road safety while driving or enhancing the reliability of eyewitness testimonies.
If you liked this article, then please subscribe to our YouTube Channel for the latest Science & Tech news. You can also find us on Twitter & Facebook