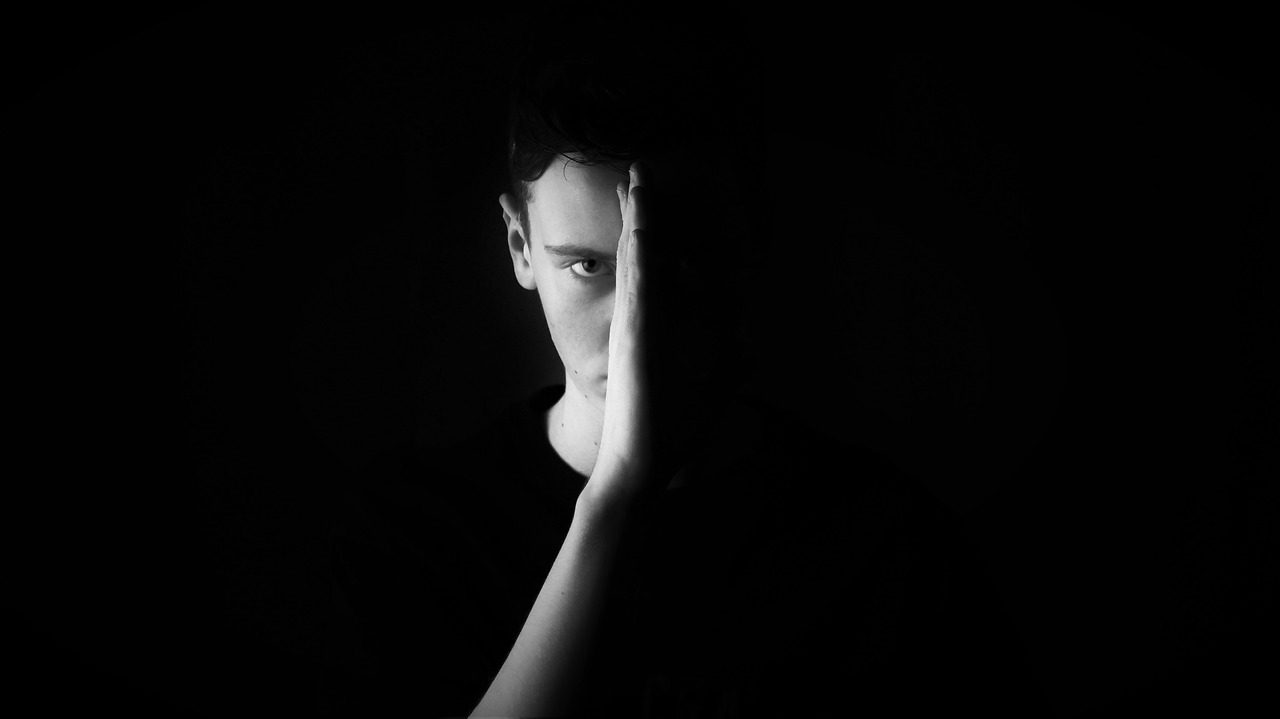
Artificial Networks Shed Light on Human Face Recognition
- News
- 1.7K
Our brains are so primed to recognize faces – or to tell people apart – that we rarely even stop to think about it, but what happens in the brain when it engages in such recognition is still far from understood.
In a new study reported today in Nature Communications, researchers at the Weizmann Institute of Science have shed new light on this issue. They found a striking similarity between the way in which faces are encoded in the brain and in successfully performing artificial intelligence systems known as deep neural networks.
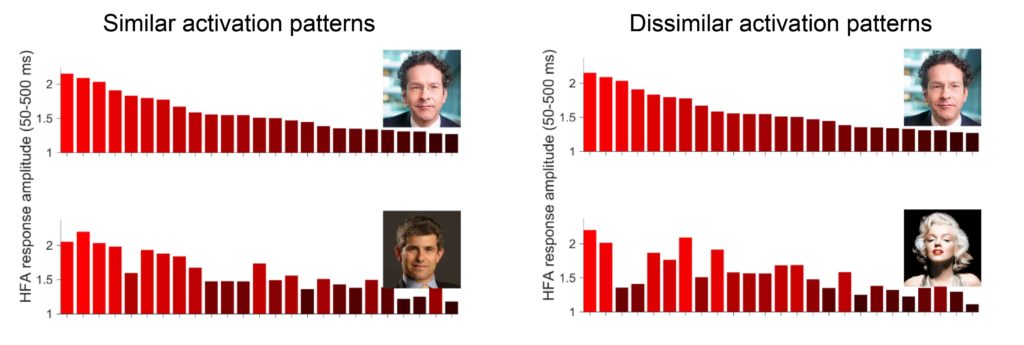
When we look at a face, groups of neurons in the visual cortex are activated and fire their signals. In fact, certain groups of neurons respond selectively to faces but not to other objects. But how does the activation of individual neurons come together to produce face perception and recognition?
Prof. Rafi Malach, of the Neurobiology Department, and Shany Grossman, a Ph.D. student in his group, had the idea of addressing this question by comparing human brain activity with deep neural networks. These computing systems, which recently revolutionized the field of artificial intelligence, are trained to perform tasks by learning from enormous data sets. In the past few years, they have improved so dramatically that they now perform as well as humans, or even better, on a variety of visual tasks, including face recognition.
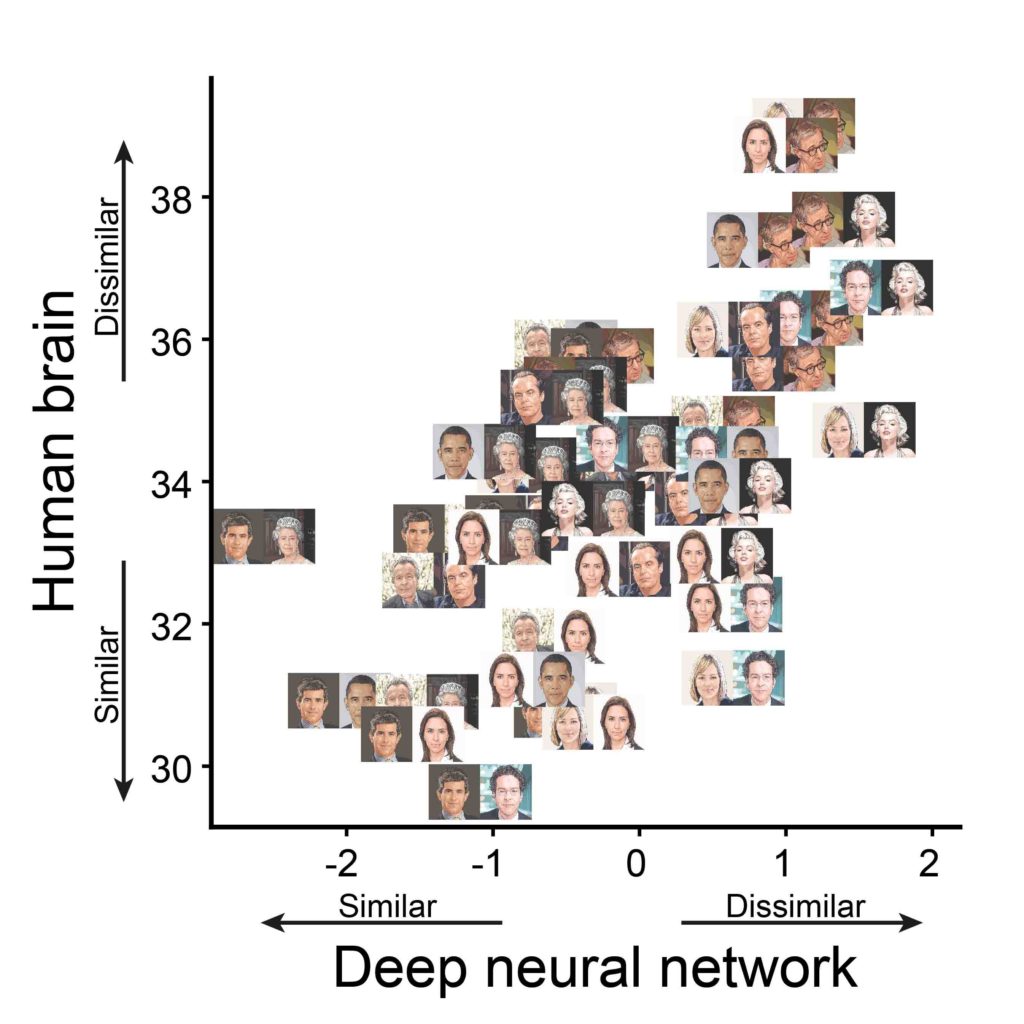
Grossman and Guy Gaziv, a research student in the Computer Science and Applied Mathematics Department, analyzed data obtained from 33 individuals in the lab of Dr. Ashesh Mehta in the Feinstein Institute for Medical Research in Manhasset, New York. This unique set of subjects are epilepsy patients who had had electrodes implanted in various regions of their brains for the purpose of diagnosis, and who volunteered to participate in various research tasks.
As the volunteers were shown a series of faces from different image databases, including famous and unfamiliar individuals, their brain activity was monitored via recordings from 96 electrodes implanted into the part of the brain responsible for face perception. The recordings showed that each face evoked a unique pattern of neuronal activation, involving different groups of neurons that fired at different intensities. Interestingly, some pairs of faces elicited similarly-looking brain activity patterns – that is, they had similar activity “signatures” – whereas others elicited activation patterns that differed greatly from one another. The researchers were curious to know whether these activation signatures play an important role in our ability to recognize faces.
They decided to compare the human face recognition system with that of a deep neural network having similar face recognition capability. This artificial network, loosely inspired by the human visual system, contains artificial elements, analogous to neurons, arranged in some two dozen “layers.” To recognize a person’s face, the artificial neurons in each layer select and combine different facial features – from the simplest ones such as lines and primitive shapes, through more complex ones such as parts of the eye and other facial fragments, to such definitive ones as a person’s identity.
The researchers reasoned that if the face-coding patterns they found in the human brain were critical for allowing humans to recognize faces, such signatures should also be found in the artificial network. To test if this was the case, they presented to the network the same images of faces shown to the human volunteers. They then checked if these faces elicited sets of a face- exclusive activation patterns that had the same diversity and structure like the ones that had been detected in the human brains.
Intriguingly, the scientists found a striking parallel between the human and artificial systems. It was most prominent in the middle layers of the deep network – those that represent the actual pictorial appearance of the faces rather than the more abstract personal identity of the face owners.
“It’s highly informative that two such drastically different systems – a biological and an artificial one, that is, the brain and a deep neural network – have evolved in such a way that they possess similar characteristics,” says Malach. “I would call this convergent evolution – just as man-made airplanes show similarity to those of wings of insects, birds, and even mammals. Such convergence points to the crucial importance of unique face-coding patterns in face recognition.”
“Our findings support the hypothesis that distinct activation patterns of neurons in response to different faces, as well as the relationship between these patterns, play a key role in the way the brain perceives faces,” says Grossman. “These findings can help advance our understanding of how face perception and recognition are encoded in the human brain. On the other hand, they may also help to further improve the performance of neural networks, by tweaking them so as to bring them closer to the observed brain response patterns.”
Study authors also included Michal Harel of the Neurobiology Department, Prof. Michal Irani of the Computer Science and Applied Mathematics Department, and Dr. Mehta’s group at the Feinstein Institute for Medical Research.
Prof. Rafi Malach’s research is supported by the Barbara and Morris L. Levinson Professorial Chair in Brain Research; the Dr. Lou Siminovitch Laboratory for Research in Neurobiology; and the estate of Florence and Charles Cuevas.
The Weizmann Institute of Science in Rehovot, Israel, is one of the world’s top-ranking multidisciplinary research institutions. Noted for its wide-ranging exploration of the natural and exact sciences, the Institute is home to scientists, students, technicians and supporting staff. Institute research efforts include the search for new ways of fighting disease and hunger, examining leading questions in mathematics and computer science, probing the physics of matter and the universe, creating novel materials and developing new strategies for protecting the environment.
If you liked this article, then please subscribe to our YouTube Channel for the latest Science & Tech news. You can also find us on Twitter & Facebook.