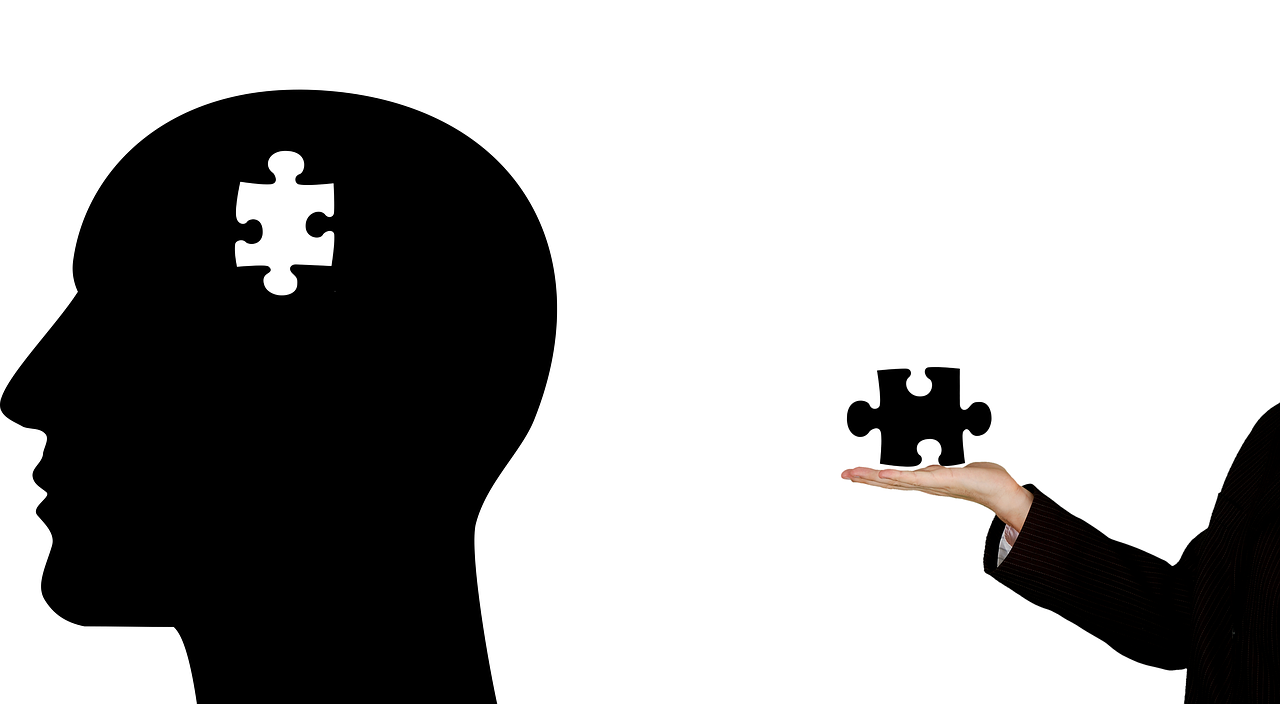
How AI May Help Diagnose Mental Illnesses
- News
- 2.1K
Artificial intelligence is finding new applications in a range of fields. Now researchers from India and Canada have developed a machine learning-based tool that can diagnose schizophrenia with high accuracy.
Although research on major psychiatric illnesses has been going on for decades, there are still no reliable methods to predict and diagnose many ailments. One of the reasons is the inherent variability in biological systems. Schizophrenia is a debilitating psychotic illness where the diagnosis is often difficult due to its numerous clinical forms and considerable overlap with other psychiatric disorders.
Researchers at the National Institute of Mental Health and Neurosciences (NIMHANS) used functional MRI (fMRI), a method in which magnetic field is used to map and measure brain activity. With this, they measured brain activity in 93 healthy and 81 schizophrenia patients.
Most previous studies had smaller groups of people who may not capture variabilities in the symptoms. In addition, patients were already undergoing therapy and taking anti-psychotic drugs that are known to alter brain activity. In the new study, patients who had not been exposed to drugs were included. This reduced the possibility of errors due to the effects of drugs.
Brain information was obtained from fMRI during the resting stage. Researchers divided the whole brain into different regions or parcels. This was done in 14 different ways based on similarities in volume, surface, connectivity etc. From each method of dividing the brain, information was derived on three features based on the region and three features based on connectivity of the brain. These parameters included the frequency of brain waves, the correlation between the brain activity of closely-placed regions, and connectivity between different brain regions. These features were chosen as previous studies show they are altered in a schizophrenic brain.
This helped researchers collate 84 points of data (from 14 brain division schemes, and 6 features extracted from each scheme) from each subject. Using these data points from healthy and schizophrenic patients, the group has built a model that could predict schizophrenia with an accuracy of 87%. The model has been named “EMPaSchiz” or ‘Ensemble algorithm with Multiple Parcellations for Schizophrenia prediction’.
“The classification accuracy our model outperforms earlier machine learning models built for diagnosing schizophrenia using resting-state fMRI on large samples,” said Ganesan Venkatasubramanian, a member of the research team, while speaking to India Science Wire.
More research is needed on the model before user-friendly software can be generated, he added. He hoped that such automated and semi-automated diagnostic tools could be developed for detecting other kinds of mental disorders and help predict treatment strategies.
The research team included Rimjhim Agrawal, Venkataram Shivakumar, Janardhanan C. Narayanaswamy, and Ganesan Venkatasubramanian (NIMHANS); Sunil Vasu Kalmady, Matthew R. G. Brown, Andrew J Greenshaw, Serdar M Dursun, Russell Greiner (Alberta Machine Intelligence Institute, University of Alberta). This study has been published in the journal Schizophrenia. (India Science Wire)
By Dr. P Surat
Journal Article
If you liked this article, then please subscribe to our YouTube Channel for the latest Science & Tech news. You can also find us on Twitter & Facebook.